Exploring spatial relationships with data.
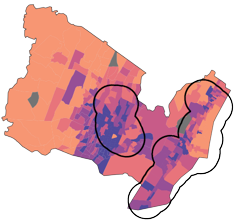
Transit Oriented Development Analysis: New Jersey
As cities and counties across the country see an influx of population growth, developing rich transit communities that are connected, accessible and integrated into the larger regional context, are becoming crucial when planning for the future.This memo attempts to analyze and interpret the spatial and social biases that may present themselves when doing this type of analysis and persuade councilmen from Essex and Hudson county on why better, quantitative and qualitative data is needed to better understand the residents’ transit values and needs.
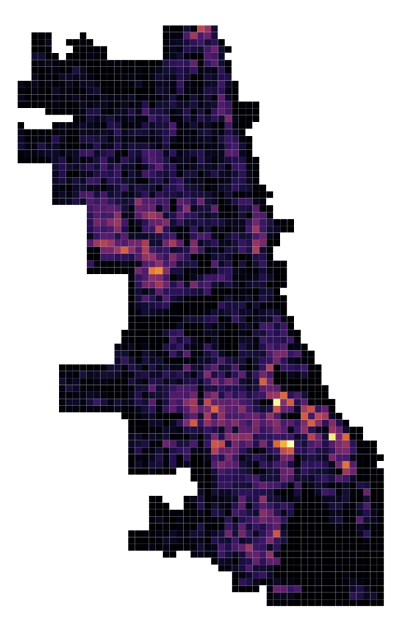
Geospatial Risk Prediction in Chicago
This project required me to build a model for a different outcome that likely suffers from more selection bias than burglary.
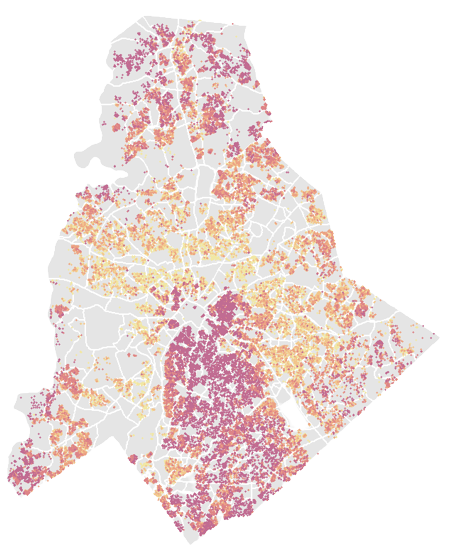
Predicting Housing Prices in Mecklenburg, NC
This project required several machine learning processes for us to better understand the housing data, the variables within the housing data, and any external data sources we decided to add. Data wrangling, exploratory analysis, feature engineering, feature selection, and model estimation and validation were all machine learning processes used as major steps in this predictive modeling exercise.
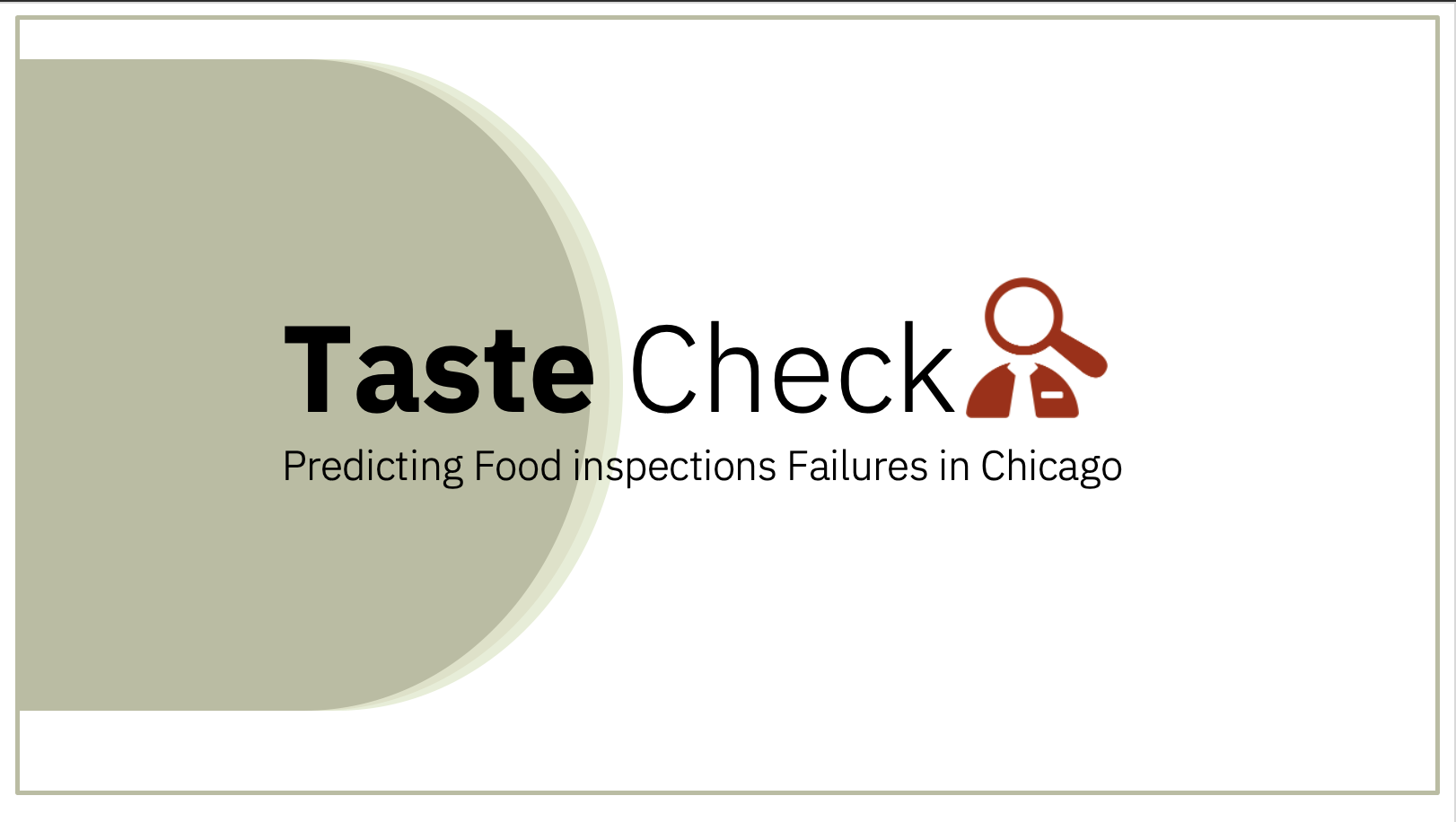
Predicting food inspection failures in Chicago
The following markdown demonstrates how we arrived at a better solution for the Chicago Public Health Departments using feature engineering, logistic regression modeling, and an efficient optimization cost-benefit analysis for efficient resource allocation.